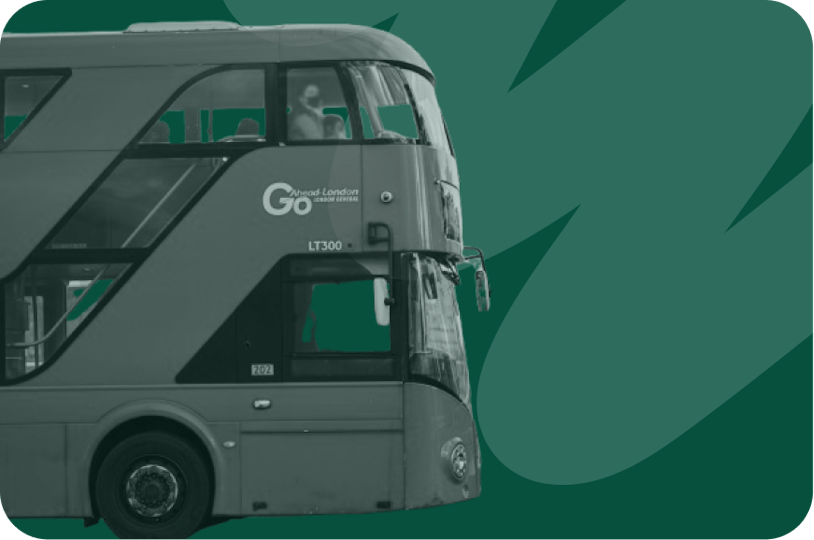
The UK’s Transport Decarbonisation Plan: Everything you need to know
In this article, we’ll unpack the key details of the UK’s Transport Decarbonisation Plan and what it means for the transport industry.
ESG / CSR
Industries
Artificial intelligence (AI) has exploded in recent years, making its way into everyday life and promising to reshape the job market. Whether it's AI chatbots like ChatGPT answering customer service queries, generative AI designing graphics and music, or AI-driven algorithms predicting financial trends, this technology is here to stay.
AI is growing at a breakneck pace:
What’s fueling this explosion? Three main factors: cheaper computing power, better machine learning models, and companies realising that AI isn’t just an experiment - it’s a necessity.
On one hand, AI is automating tasks, cutting down on repetitive work, and streamlining operations. However, many administrative and data-processing roles are at risk. The International Monetary Fund estimates that AI could impact 40% of jobs worldwide, with some roles disappearing entirely.
On the other hand, AI isn’t just eliminating jobs - it’s creating new ones. Entire fields like AI ethics, AI auditing, and machine learning engineering didn’t exist a decade ago. Many companies are hiring AI specialists at record rates, and workers who learn how to use AI effectively are becoming more valuable.
Then there's the "AI agent" revolution. Businesses are increasingly using AI-powered digital workers - virtual assistants that handle emails, schedule meetings, write reports, and even analyse market trends.
Some examples?
Despite its widespread use, public awareness of AI’s presence in everyday life remains mixed. According to a Pew Research Center survey of 11,004 adults in the US, 27 % of respondents say they interact with AI several times a day, while another 28 % believe they use it about once a day or several times a week. However, 44 % of respondents think they do not regularly interact with AI at all.
This perception gap suggests that AI’s role is far more extensive than people realise. In the same survey, only 30 % of Americans could correctly identify six common examples of AI in daily life. This means that while many people report using AI sparingly, they likely interact with it far more frequently than they recognise.
AI isn't just making life more convenient, it’s reshaping industries.
Beyond its environmental impact, AI’s rapid expansion has also raised concerns about its misuse. For example, during the 2024 presidential election, Donald Trump circulated deepfake videos of Taylor Swift, potentially misleading fans into believing she had endorsed him. As AI-generated content becomes more advanced, addressing the ethical risks - and the additional energy demands of training and running these models - will be increasingly critical.
For all its benefits, AI comes at a high environmental price.
AI’s resource consumption is only set to grow as competition in the sector accelerates. In the US a $500 billion private-sector investment led by OpenAI, SoftBank, and Oracle aims to expand AI infrastructure with the construction of new data centers, intensifying concerns over energy and water use. Meanwhile, China’s DeepSeek launch has disrupted the market, signaling an escalating global AI race with mounting environmental consequences.
Among the many AI applications reshaping our world, one stands out in both its impact and scale - ChatGPT. With hundreds of millions of users and a rapidly growing presence in daily workflows, AI-powered chatbots are driving up energy demand like never before.
The environmental cost of these systems is particularly striking, as the power required to generate text, answer queries, and sustain ongoing conversations adds up to an enormous carbon footprint. ChatGPT, one of the most widely used AI models, offers a case study in just how energy-intensive AI has become.
By December 2024, ChatGPT had reached an astounding 300 million users - a number that continues to grow as AI becomes further embedded in daily life.
Yet, while AI adoption skyrockets, the environmental impact of ChatGPT remains largely unknown. Data centers are projected to expand by 28 % by 2030, and AI’s energy demands are rapidly rising, with estimates suggesting it could account for 3–4 % of global electricity consumption by the end of the decade. Carbon emissions linked to AI are also expected to double between 2022 and 2030, amplifying its environmental footprint.
The previous iteration of ChatGPT, ChatGPT-3, with 175 billion parameters, consumed 1,287 MWh of electricity per year - equivalent to 502 metric tons of carbon emissions, or the annual footprint of 112 gasoline-powered cars!
By 2025, ChatGPT is more relevant than ever, with users relying on AI for everything from planning vacations to handling tedious work emails.
However, as AI becomes more sophisticated, the need for multi-agent systems (where multiple AI models work together to complete complex tasks) will grow. This shift could significantly increase computing demands and energy consumption, ultimately raising AI’s overall carbon footprint.
So, how is ChatGPT shaping daily life in 2025?
As ChatGPT’s role in daily life expands, so too do questions about its environmental impact, ethical use, and long-term implications for human interaction.
Take a simple task like using AI to draft a routine email. While writing in one language may seem inconsequential, ChatGPT relies heavily on GPUs (Graphics Processing Units) for both training and inference, given their high parallel processing capabilities - making even simple AI interactions surprisingly energy-intensive.
As a result, generating a single AI response consumes roughly the same amount of energy as fully charging a smartphone. This highlights how even casual AI use contributes to excess carbon emissions - especially at scale.
A study, conducted by Hugging Face and Carnegie Mellon University and led by Sasha Luccioni, an AI researcher at Hugging Face, evaluated 88 different AI models performing popular tasks such as:
The table below shows the study's results for the energy consumption of different AI tasks.
AI Task | Energy Used per 1,000 Queries (kWh) | CO₂ Emissions per 1,000 Queries |
---|---|---|
Text classification | 0.002 | ~0.3g CO₂e |
Text generation | 0.047 | ~7.5g CO₂e |
Summarization | 0.049 | ~8g CO₂e |
Image classification | 0.007 | ~1.1g CO₂e |
Object detection | 0.038 | ~6.1g CO₂e |
Image generation | 2.9 | 1,594g CO₂e (4.1 miles driven) |
The results from the study highlight the significant energy demands of generative AI compared to task-oriented AI.
Additional research supports these findings, showing that generating 1,000 images can produce as much CO₂ as driving 4.1 miles (6.6 km) in a gasoline-powered car. In contrast, text generation requires far less energy - consuming only 16 % of a full smartphone charge, with emissions equivalent to driving just 0.0006 miles.
On average, studies have found that AI image generation is 60 times more energy-intensive than text generation.
Beyond inference, generative AI models also require extensive training, further increasing their overall energy footprint. For example, training the BLOOMz AI model (with over 100 billion parameters) consumes 0.0001 kWh per query. To put this into perspective, even the least energy-efficient image-generation model used as much energy as 522 smartphone charges for just 1,000 inferences.
Overall, the shift to AI agent networks and expanded generative AI use is expected to drive a significant increase in energy consumption, placing greater demand on data centers and raising concerns about the environmental impact of AI development.
But just how big is this impact? To quantify AI’s environmental toll, we need to break down the carbon footprint of one of the most widely used AI models - ChatGPT4.
Before we dig into the numbers, let's first take a look at the difference between GPT-4 and ChatGPT:
While ChatGPT and GPT-4 are often mentioned interchangeably, they serve distinct purposes:
In essence, GPT-4 is the foundation, while ChatGPT is a tailored implementation designed to enhance dialogue-based AI experiences.
To assess the carbon emissions associated with GPT-4, Greenly has modeled a realistic business use case: automatically replying to 1 million emails per month over the course of a year. This scenario captures the emissions generated across two key stages: training and use, both of which contribute to GPT-4’s overall carbon footprint.
For training, this estimate assumes that 25,000 NVIDIA A100 GPUs were used over a 100-day period at 30 % workload. The data centers are modeled as large-scale, well-optimised facilities with a Power Usage Effectiveness (PUE) of 1.1, located in the United States, where the average electricity mix emits 403.6 gCO₂e/kWh (based on IEA data).
By considering both the training and usage phases, this methodology provides a comprehensive view of GPT-4’s carbon impact in a real-world business application.
GPT-4 first needs to be trained to become competent and efficient at writing appropriate email responses - a process that requires extensive computing power over many hours. In this scenario, as per our methodology, we assume the training occurs in large-scale data centers with a Power Usage Effectiveness (PUE) of 1.1.
Training GPT-4 in a data center requires a range of equipment, including servers, cooling systems, and networking infrastructure. Rather than accounting for the continuous operation of this equipment over a full year, the environmental impact is calculated by amortising the energy consumption from the 100-day training period over one year. This results in a total of 5,759,430 kgCO₂e (kilograms of carbon dioxide equivalent).
In addition to direct energy consumption, data centers also contribute to emissions through refrigerant leaks from cooling systems, which are essential for preventing overheating in high-performance computing environments. Over the course of a year, these refrigerant leaks are estimated to produce an additional 49,587 kgCO₂e.
The manufacturing of GPUs used to train GPT-4 also carries a significant footprint, producing and estimated 1,329,141 kgCO₂e (based on a 4-year lifespan and 30% workload).
The total emissions from training GPT-4 over one year amount to 7,138,158 kgCO₂e.
Once GPT-4 has been fully trained, it can be deployed to perform the task of automatically responding to 1 million emails per month. Over the course of a year, this amounts to 12 million emails, with the following carbon emissions.
Factoring in electricity consumption, cooling requirements, and server manufacturing, the operation of GPT-4 for this task results in a total of 514,800 kgCO₂e over one year - equivalent to 42,900 kgCO₂e per month.
It should also be noted that if GPT-4 were to be used to respond to emails in more than one language, it would be necessary to re-train the technology for each language. This means that total emissions scale with the number of languages required, making the carbon footprint significant for companies operating in multiple languages.
However, the impact can be mitigated by adjusting the training frequency or leveraging the model for multiple tasks, which helps amortise its overall environmental footprint over a broader range of applications.
This is where emerging players like DeepSeek are stepping in, claiming to deliver comparable AI capabilities with a fraction of the computing power. Could this signal a shift toward more sustainable AI development? If DeepSeek’s approach proves scalable, it may offer a glimpse into a future where AI innovation and environmental responsibility go hand in hand.
On the 20th of January, China’s DeepSeek sent shockwaves through the AI industry, promising a breakthrough that could dramatically reduce the energy footprint of artificial intelligence. The company claims its R1 model achieves performance comparable to OpenAI’s GPT-4 while using a fraction of the computing power.
Unlike its American rivals, which rely on thousands of high-powered Nvidia chips, DeepSeek claims to have trained its model using just 2,000 Nvidia H800 chips - a fraction of the 16,000+ chips needed for comparable models like Meta’s Llama 3.1. The result? Training DeepSeek R1 reportedly cost just $6 million, compared to an estimated $60 million for Meta’s model.
At first glance, this efficiency breakthrough appears to be an environmental game-changer. AI’s growing energy demands have raised alarms, with projections suggesting that data centers could account for up to 12 % of US electricity consumption by 2028, up from 4.4 % in 2023 - a similar trend can be expected in other technology-reliant countries around the world.
Globally, AI infrastructure is already responsible for roughly 1% of energy-related greenhouse gas emissions, with the International Energy Agency (IEA) warning that global electricity demand for AI and data centers could double by next year, reaching levels comparable to Japan’s entire annual electricity consumption.
A key innovation behind this efficiency is DeepSeek’s Mixture-of-Experts (MoE) architecture. Unlike conventional AI models that activate their full network for every query, MoE assigns tasks to specialised sub-models, only activating the necessary computing power for a given request. This selective computation reduces overall energy consumption while maintaining performance.
Additionally, DeepSeek claims its model is more efficient in data storage and does not require the same level of high-performance computing hardware, helping to further reduce resource demand.
The company had to develop these innovations out of necessity - due to US sanctions restricting access to Nvidia’s most advanced AI chips. This limitation forced DeepSeek to design models that maximise efficiency rather than relying on large-scale computing power.
If DeepSeek’s claims hold true, its approach could significantly lower the emissions associated with AI. Some key advantages include:
While DeepSeek’s efficiency breakthroughs are promising, they also raise concerns that this increased efficiency will lead to higher overall consumption. Microsoft CEO Satya Nadella acknowledged this risk, posting on X, “Jevons paradox strikes again! As AI gets more efficient and accessible, we will see its use skyrocket, turning it into a commodity we just can’t get enough of.”
If AI models become cheaper and more efficient, adoption could grow exponentially, ultimately increasing electricity demand rather than reducing it.
However, it remains to be seen whether DeepSeek’s efficiency claims will translate into meaningful reductions in global AI emissions.
For AI to become truly sustainable, improvements in model efficiency must be paired with responsible deployment and investments in clean energy infrastructure. Otherwise, AI’s environmental footprint will continue to grow, even as individual models become more efficient.
As AI adoption accelerates, so does its environmental impact. However, there are several ways to reduce the energy intensity of generative AI:
While AI can improve efficiency, its energy demands must be managed carefully. Proactive strategies like these will be essential to making AI’s future more sustainable.